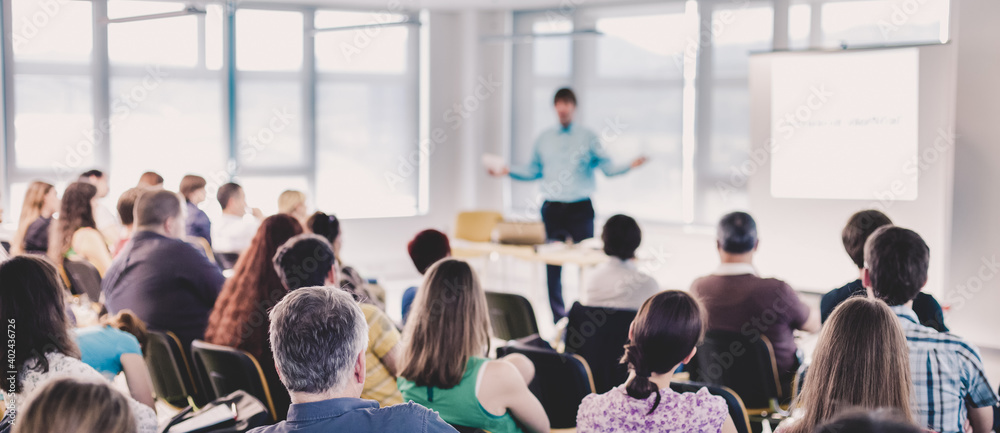
Denisa BANULESCU-RADU
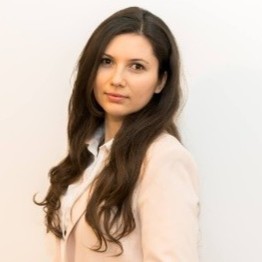
BANULESCU-RADU
Denisa
e
Domaine de recherche : Économétrie
Bureau : A212
E-mail : georgiana-denisa.radu@univ-orleans.fr
Site internet : Page personnelle
Travaux
- Publications dans des revues scientifiques
- Ouvrages et rapports
- Documents de travail et autres publications
- Communications
2023
Practical guideline to efficiently detect insurance fraud in the era of machine learning: A household insurance case
Abstract Identifying insurance fraud is a difficult task due to the complex nature of the fraud itself, the diversity of techniques employed, the rarity of fraud cases observed in data sets, and the relatively limited allocation of human, financial, and time resources to carry out investigations. The aim of this paper is to provide a clean and well structured study on modeling fraud on home insurance contracts, using real French data from 2013 to 2017. Several methods are developed to identify risk factors and unusual customer behaviors. Traditional econometric models as well as new machine‐learning algorithms with good predictive performance and high operational efficiency are tested, while maintaining method interpretability. Each methodology is evaluated on the basis of adequate performance measures and the issue of imbalanced databases is also addressed. Finally, specific methods are applied to interpret the results of the machine‐learning methods.
Lien HAL2021
Backtesting Marginal Expected Shortfall and Related Systemic Risk Measures
This paper proposes an original approach for backtesting systemic risk measures. This backtesting approach makes it possible to assess the systemic risk measure forecasts used to identify the financial institutions that contribute the most to the overall risk in the financial system. Our procedure is based on simple tests similar to those generally used to backtest the standard market risk measures such as value-at-risk or expected shortfall. We introduce a concept of violation associated with the marginal expected shortfall (MES), and we define unconditional coverage and independence tests for these violations. We can generalize these tests to any MES-based systemic risk measures such as the systemic expected shortfall (SES), the systemic risk measure (SRISK), or the delta conditional value-at-risk ([Formula: see text]CoVaR). We study their asymptotic properties in the presence of estimation risk and investigate their finite sample performance via Monte Carlo simulations. An empirical application to a panel of U.S. financial institutions is conducted to assess the validity of MES, SRISK, and [Formula: see text]CoVaR forecasts issued from a bivariate GARCH model with a dynamic conditional correlation structure. Our results show that this model provides valid forecasts for MES and SRISK when considering a medium-term horizon. Finally, we propose an early warning system indicator for future systemic crises deduced from these backtests. Our indicator quantifies how much is the measurement error issued by a systemic risk forecast at a given point in time which can serve for the early detection of global market reversals. This paper was accepted by Kay Giesecke, finance.
Lien HAL2016
Do We Need High Frequency Data to Forecast Variances?
In this paper we study various MIDAS models for which the future daily variance is directly related to past observations of intraday predictors. Our goal is to determine if there exists an optimal sampling frequency in terms of variance prediction. Via Monte Carlo simulations we show that in a world without microstructure noise, the best model is the one using the highest available frequency for the predictors. However, in the presence of microstructure noise, the use of very high-frequency predictors may be problematic, leading to poor variance forecasts. The empirical application focuses on two highly liquid assets (i.e., Microsoft and S&P 500). We show that, when using raw intraday squared log-returns for the explanatory variable, there is a “high-frequency wall” – or frequency limit – above which MIDAS-RV forecasts deteriorate or stop improving. An improvement can be obtained when using intraday squared log-returns sampled at a higher frequency, provided they are pre-filtered to account for the presence of jumps, intraday diurnal pattern and/or microstructure noise. Finally, we compare the MIDAS model to other competing variance models including GARCH, GAS, HAR-RV and HAR-RV-J models. We find that the MIDAS model – when it is applied on filtered data –provides equivalent or even better variance forecasts than these models. JEL: C22, C53, G12 / KEY WORDS: Variance Forecasting, MIDAS, High-Frequency Data. RÉSUMÉ. Nous considérons dans cet article des modèles de régression MIDAS pour examiner l'influence de la fréquence d'échantillonnage des prédicteurs sur la qualité des prévisions de la volatilité quotidienne. L'objectif principal est de vérifier si l'information incorporée par les prédicteurs à haute fréquence améliore la qualité des précisions de volatilité, et si oui, s'il existe une fréquence d'échantillonnage optimale de ces prédicteurs en termes de prédiction de la variance. Nous montrons, via des simulations Monte Carlo, que dans un monde sans bruit de microstructure, le meilleur modèle est celui qui utilise des prédicteurs à la fréquence la plus élevée possible. Cependant, en présence de bruit de microstructure, l'utilisation des měmes prédicteurs à haute fréquence peut ětre problématique, conduisant à des prévisions pauvres de la variance. L'application empirique se concentre sur deux actifs très liquides (Microsoft et S & P 500). Nous montrons que, lors de l'utilisation des rendements intra-journaliers au carré pour la variable explicative, il y a un « mur à haute fréquence » – ou limite de fréquence – au-delà duquel les prévisions des modèles MIDAS-RV se détériorent ou arrětent de s'améliorer. Une amélioration pourrait ětre obtenue lors de l'utilisation des rendements au carré échantillonnés à une fréquence plus élevée, à condition qu'ils soient préfiltrés pour tenir compte de la présence des sauts, de la saisonnalité intra-journalière et/ou du bruit de microstructure. Enfin, nous comparons le modèle MIDAS à d'autres modèles de variance concurrents, y compris les modèles GARCH, GAS, HAR-RV et HAR-RV-J. Nous constatons que le modèle MIDAS – quand il est appliqué sur des données filtrées – fournit des prévisions de variance équivalentes ou měme meilleures que ces modèles.
Lien HAL2015
2019
2020
Backtesting Marginal Expected Shortfall and Related Systemic Risk Measures
Résumé non disponible.
Lien HAL2019
Prévoir la volatilité d’un actif financier à l’aide d’un modèle à mélange de fréquences
Résumé non disponible.
Lien HAL2014
Do We Need Ultra-High Frequency Data to Forecast Variances?
In this paper we study various MIDAS models in which the future daily variance is directly related to past observations of intraday predictors. Our goal is to determine if there exists an optimal sampling frequency in terms of volatility prediction. Via Monte Carlo simulations we show that in a world without microstructure noise, the best model is the one using the highest available frequency for the predictors. However, in the presence of microstructure noise, the use of ultra high-frequency predictors may be problematic, leading to poor volatility forecasts. In the application, we consider two highly liquid assets (i.e., Microsoft and S&P 500). We show that, when using raw intraday squared log-returns for the explanatory variable, there is a "high-frequency wall" or frequency limit above which MIDAS-RV forecasts deteriorate. We also show that an improvement can be obtained when using intraday squared log-returns sampled at a higher frequency, provided they are pre-filtered to account for the presence of jumps, intraday periodicity and/or microstructure noise. Finally, we compare the MIDAS model to other competing variance models including GARCH, GAS, HAR-RV and HAR-RV-J models. We find that the MIDAS model provides equivalent or even better variance forecasts than these models, when it is applied on filtered data.
Lien HAL2013
High-Frequency Risk Measures
This paper proposes intraday High Frequency Risk (HFR) measures for market risk in the case of irregularly spaced high-frequency data. In this context, we distinguish three concepts of value-at-risk (VaR): the total VaR, the marginal (or per-time-unit) VaR, and the instantaneous VaR. Since the market risk is obviously related to the duration between two consecutive trades, these measures are completed with a duration risk measure, i.e., the time-at-risk (TaR). We propose a forecasting procedure for VaR and TaR for each trade or other market microstructure event. We perform a backtesting procedure specifically designed to assess the validity of the VaR and TaR forecasts on irregularly spaced data. The performance of the HFR measure is illustrated in an empirical application for two stocks (Bank of America and Microsoft) and an exchange-traded fund (ETF) based on Standard and Poor's (the S&P) 500 index. We show that the intraday HFR forecasts accurately capture the volatility and duration dynamics for these three assets.
Lien HALAucune publication disponible pour le moment.