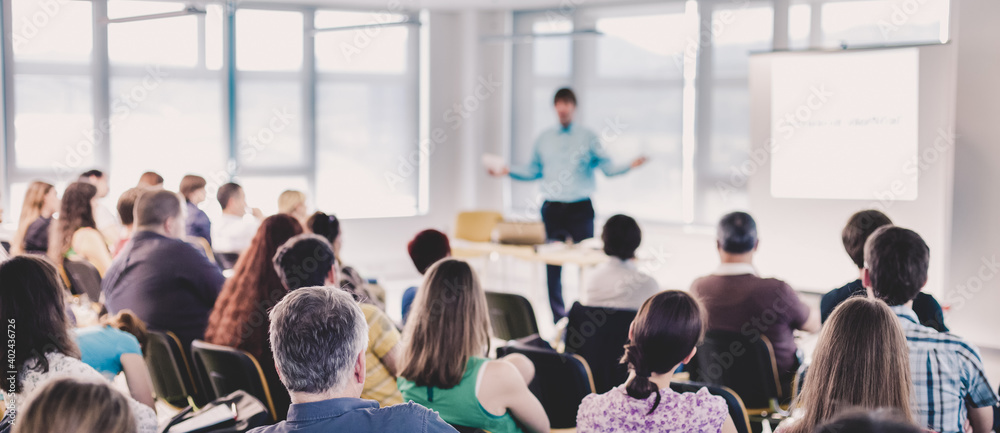
Sessi TOKPAVI
TOKPAVI
Sessi
enseignant-chercheurs
Domaine de recherche : Économétrie
Bureau : A213
E-mail : sessi.tokpavi@univ-orleans.fr
Site internet : Page personnelle
Travaux
- Publications dans des revues scientifiques
- Ouvrages et rapports
- Documents de travail et autres publications
- Communications
2022
Machine Learning for Credit Scoring: Improving Logistic Regression with Non Linear Decision Tree Effects
In the context of credit scoring, ensemble methods based on decision trees, such as the random forest method, provide better classification performance than standard logistic regression models. However, logistic regression remains the benchmark in the credit risk industry mainly because the lack of interpretability of ensemble methods is incompatible with the requirements of financial regulators. In this paper, we propose a high-performance and interpretable credit scoring method called penalised logistic tree regression (PLTR), which uses information from decision trees to improve the performance of logistic regression. Formally, rules extracted from various short-depth decision trees built with original predictive variables are used as predictors in a penalised logistic regression model. PLTR allows us to capture non-linear effects that can arise in credit scoring data while preserving the intrinsic interpretability of the logistic regression model. Monte Carlo simulations and empirical applications using four real credit default datasets show that PLTR predicts credit risk significantly more accurately than logistic regression and compares competitively to the random forest method
Lien HAL2019
Measuring network systemic risk contributions: A leave-one-out approach
Résumé non disponible.
Lien HAL2017
Quand l’union fait la force : un indice de risque systémique, When unity makes strenght: a systemic risk index
À la suite de la dernière crise financière sévère, plusieurs mesures de risque systémique ont été proposées pour quantifier l’état de stress du système financier. Dans cet article, nous proposons un indice agrégé de mesure de risque systémique financier basé sur une analyse en composantes principales dite « parcimonieuse ». Cette méthodologie permet d’obtenir un indice agrégé plus parcimonieux et plus stable dans le temps. L’application de la méthodologie à douze mesures de risque systémique global en utilisant des données des titres du marché financier américain confirme cette propriété. Il apparaît par ailleurs que les mouvements extrêmes positifs de l’indice de risque systémique ainsi construit peuvent être considérés comme des anticipations des périodes de forte contraction de l’activité économique. In the aftermath of the last severe financial crisis, several systemic risk measures have been proposed in the literature for quantifying financial system-wide distress. In this article, we propose an aggregated index for financial systemic risk measurement based on Sparse Principal Component Analysis. This methodology helps to obtain an index with more stable time dynamics. The results obtained using financial US market data confirm the temporal stability property. It appears, finally, that positive extreme movements of the proposed Index of Systemic Risk Measures are leading indicators of periods of sharp economic downturn.
Lien HAL2016
2007
Une évaluation des procédures de Backtesting : Tout va pour le mieux dans le meilleur des mondes
Résumé non disponible.
Lien HALUne évaluation des procédures de Backtesting : Tout va pour le mieux dans le meilleur des mondes
Résumé non disponible.
Lien HAL2006
Aucune publication disponible pour le moment.
2023
Are ESG ratings informative to forecast idiosyncratic risk?
Sustainable investing is growing fast and investors are increasingly integrating environmental, social, and governance (ESG) criteria. However, ESG ratings are derived using heterogeneous methodologies and can be quite divergent across providers, which suggests the need for a formal statistical procedure to evaluate their accuracy. This paper develops a backtesting procedure that evaluates how well these extra-financial metrics help in predicting a company's idiosyncratic risk. Technically, the inference is based on extending the conditional predictive ability test of Giacomini and White (2006) to a panel data setting. We apply our methodology to the forecasting of stock returns idiosyncratic volatility and compare two ESG rating systems from Sustainalytics and Asset4 across three investment universes (Europe, North America, and the Asia-Pacific region). The results show that the null hypothesis of no informational content in ESG ratings is strongly rejected in Europe, whereas results appear mixed in the other regions. Furthermore, the predictive accuracy gains are higher when considering the environmental dimension of ESG ratings. Importantly, applying the test only to firms over which there is a high degree of consensus between the ESG rating agencies leads to higher predictive accuracy gains for all three universes. Beyond providing insights into the accuracy of each of the ESG rating systems, this last result suggests that information gathered from several ESG rating providers should be cross-checked before ESG is integrated into investment processes.
Lien HAL2021
Machine Learning or Econometrics for Credit Scoring: Let's Get the Best of Both Worlds
In the context of credit scoring, ensemble methods based on decision trees, such as the random forest method, provide better classification performance than standard logistic regression models. However, logistic regression remains the benchmark in the credit risk industry mainly because the lack of interpretability of ensemble methods is incompatible with the requirements of financial regulators. In this paper, we pro- pose to obtain the best of both worlds by introducing a high-performance and interpretable credit scoring method called penalised logistic tree regression (PLTR), which uses information from decision trees to improve the performance of logistic regression. Formally, rules extracted from various short-depth decision trees built with pairs of predictive variables are used as predictors in a penalised logistic regression model. PLTR allows us to capture non-linear effects that can arise in credit scoring data while preserving the intrinsic interpretability of the logistic regression model. Monte Carlo simulations and empirical applications using four real credit default datasets show that PLTR predicts credit risk significantly more accurately than logistic regression and compares competitively to the random forest method. JEL Classification: G10 C25, C53
Lien HAL2013
High-Frequency Risk Measures
This paper proposes intraday High Frequency Risk (HFR) measures for market risk in the case of irregularly spaced high-frequency data. In this context, we distinguish three concepts of value-at-risk (VaR): the total VaR, the marginal (or per-time-unit) VaR, and the instantaneous VaR. Since the market risk is obviously related to the duration between two consecutive trades, these measures are completed with a duration risk measure, i.e., the time-at-risk (TaR). We propose a forecasting procedure for VaR and TaR for each trade or other market microstructure event. We perform a backtesting procedure specifically designed to assess the validity of the VaR and TaR forecasts on irregularly spaced data. The performance of the HFR measure is illustrated in an empirical application for two stocks (Bank of America and Microsoft) and an exchange-traded fund (ETF) based on Standard and Poor's (the S&P) 500 index. We show that the intraday HFR forecasts accurately capture the volatility and duration dynamics for these three assets.
Lien HAL2008
Backtesting Value-at-Risk: A GMM Duration-Based Test
This paper proposes a new duration-based backtesting procedure for VaR forecasts. The GMM test framework proposed by Bontemps (2006) to test for the distributional assumption (i.e. the geometric distribution) is applied to the case of the VaR forecasts validity. Using simple J-statistic based on the moments defined by the orthonormal polynomials associated with the geometric distribution, this new approach tackles most of the drawbacks usually associated to duration based backtesting procedures. First, its implementation is extremely easy. Second, it allows for a separate test for unconditional coverage, independence and conditional coverage hypothesis (Christoffersen, 1998). Third, feasibility of the tests is improved. Fourth, Monte-Carlo simulations show that for realistic sample sizes, our GMM test outperforms traditional duration based test. An empirical application for Nasdaq returns confirms that using GMM test leads to major consequences for the ex-post evaluation of the risk by regulation authorities. Without any doubt, this paper provides a strong support for the empirical application of duration-based tests for VaR forecasts.
Lien HALSélection dynamique de portefeuille dans un cadre Moyenne-VaR: une approche GARCH multivariée
Résumé non disponible.
Lien HAL2007
Irregularly Spaced Intraday Value at Risk (ISIVaR) Models : Forecasting and Predictive Abilities
The objective of this paper is to propose a market risk measure defined in price event time and a suitable backtesting procedure for irregularly spaced data. Firstly, we combine Autoregressive Conditional Duration models for price movements and a non parametric quantile estimation to derive a semi-parametric Irregularly Spaced Intraday Value at Risk (ISIVaR) model. This ISIVaR measure gives two information: the expected duration for the next price event and the related VaR. Secondly, we use a GMM approach to develop a backtest and investigate its finite sample properties through numerical Monte Carlo simulations. Finally, we propose an application to two NYSE stocks.
Lien HALUne Evaluation des Procédures de Backtesting
Dans cet article, nous proposons une démarche originale visant à évaluer la capacité des tests usuels de backtesting à discriminer différentes prévisions de Value at Risk (VaR) ne fournissant pas la même évaluation ex-ante du risque. Nos résultats montrent que, pour un même actif, ces tests conduisent très souvent à ne pas rejeter la validité, au sens de la couverture conditionnelle, de la plupart des six prévisions de VaR étudiées, même si ces dernières sont sensiblement différentes. Autrement dit, toute prévision de VaR a de fortes chances d'être validée par ce type de procédure.
Lien HALIrregularly Spaces Intraday Value-at-Risk (ISIVaR) Models: Forecasting and Predictive Abilities
Résumé non disponible.
Lien HALIrregularly Spaced Intraday Value at Risk (ISIVaR) Models: Forecasting and Predictive Abilities
Résumé non disponible.
Lien HALIrregularly Spaced Intraday Value at Risk (ISIVaR) Models Forecasting and Predictive Abilities
Résumé non disponible.
Lien HAL2006
Backtesting VaR Accuracy: A New Simple Test
This paper proposes a new test of Value at Risk (VaR) validation. Our test exploits the idea that the sequence of VaR violations (Hit function) - taking value 1-α, if there is a violation, and -α otherwise - for a nominal coverage rate α verifies the properties of a martingale difference if the model used to quantify risk is adequate (Berkowitz et al., 2005). More precisely, we use the Multivariate Portmanteau statistic of Li and McLeod (1981) - extension to the multivariate framework of the test of Box and Pierce (1970) - to jointly test the absence of autocorrelation in the vector of Hit sequences for various coverage rates considered as relevant for the management of extreme risks. We show that this shift to a multivariate dimension appreciably improves the power properties of the VaR validation test for reasonable sample sizes.
Lien HAL2008
2007
International Symposium on Financial Engineering and Risk Management 2007
Résumé non disponible.
Lien HAL2006
2005