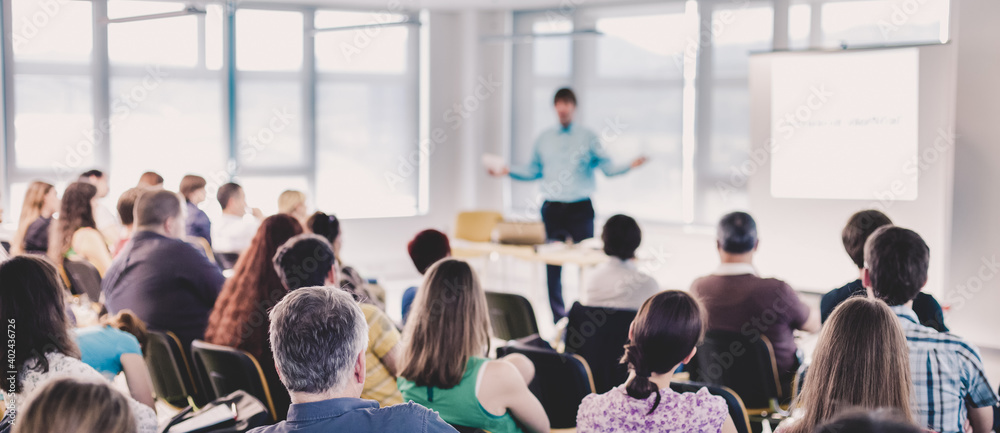
Christophe HURLIN
HURLIN
Christophe
Directeur
enseignant-chercheurs
Domaine de recherche : Économétrie
Bureau : A211
Responsabilités
- Membre senior de l’Institut Universitaire de France (IUF), 2022-
- Membre du comité scientifique de l’ACPR, 2018-
- Directeur adjoint du cascad (UAR CNRS, HEC, UO), 2017-
- Directeur du Laboratoire d’Economie d’Orléans, 2016-
- Co-responsable du Master Économétrie et Statistique Appliquée (ESA), 2004-
- Membre du comité d’évaluation scientifique CE26 de l’ANR, depuis 2021-
- Responsable de l’équipe Économétrie du LEO (2008-2016)
Encadrement doctoral
Thèses en cours
- Sébastien Saurin (2021-) : Algorithmic fairness in finance, Co-supervisor: Christophe Pérignon (HEC).
- Yannick Kougblenou (2021-) : Data Science and Machine Learning for financial fraud detection. Co-supervisor: Denisa Banulescu-Radu (University of Orléans).
Thèse Soutenues
Normal
0
21
false
false
false
FR
X-NONE
X-NONE
/* Style Definitions */
table.MsoNormalTable
{mso-style-name: »Tableau Normal »;
mso-tstyle-rowband-size:0;
mso-tstyle-colband-size:0;
mso-style-noshow:yes;
mso-style-priority:99;
mso-style-parent: » »;
mso-padding-alt:0cm 5.4pt 0cm 5.4pt;
mso-para-margin-top:0cm;
mso-para-margin-right:0cm;
mso-para-margin-bottom:10.0pt;
mso-para-margin-left:0cm;
line-height:115%;
mso-pagination:widow-orphan;
font-size:11.0pt;
font-family: »Calibri »,sans-serif;
mso-ascii-font-family:Calibri;
mso-ascii-theme-font:minor-latin;
mso-hansi-font-family:Calibri;
mso-hansi-theme-font:minor-latin;
mso-bidi-font-family: »Times New Roman »;}
- Ophélie Couperier (2017-2022). Three essays in financial econometrics“. Co-supervisor: Christian Francq (CREST) and Jean-Michel Zakoian (CREST). Current position : ATER University Paris Dauphine.
- Olessia Caillé (2016-2021): “ Risk-based investment strategies“. Co-supervisor: Daria Onori (University of Orléans). Current position: assistant professor at ISC Paris.
- Jérémy Leymarie (2015-2019). Three essays in financial econometrics. Co-supervisors: Alain Hecq (Maastricht University) and Denisa Banulescu (University of Orléans). Past position: post-doc University of Vienna. Current position: assistant professor EDHEC. Best Paper PhD prize, German Association of Finance 2019 and AFFI (French Association of Finance) Thesis Prize 2020 (market finance).
- Michael Richard (2015-2019). Evaluation and validation of density forecasts. Co-supervisor: Jérôme Collet (EDF R&D Osiris). Current position : Data Scientist, Institut Curie, INSERM.
- Denisa Banulescu (2011-2014). Three essays in financial econometrics. Co-supervisor: Bertrand Candelon (Maastricht University). Max Weber Fellowship (2014-2015) at the European University Institute (EUI, Florence). Monetary, Financial and Banking Thesis Prize 2015 of the Banque de France Foundation and Young researcher prize 2016 of the Autorité des Marchés Financiers (AMF). Current position: associate professor University of Orléans.
- Sylvain Benoit (2010-2014). Three essays on systemic risk. Co-supervisor: Christophe Pérignon (HEC, Paris). Current position: associate professor at University Paris Dauphine. SAB thesis Prize 2015 of sustainable finance.
- Elena Dumitrescu (2009-2011). Early warning systems. Co-supervisor: Bertrand Candelon (Maastricht University). Max Weber Fellowship (2011-2012) at the European University Institute (EUI, Florence). Current position: associate professor at University Paris Ouest Nanterre.
- Jaouad Madkour (2008-2012). Non-linear times series models. Co-supervisor: Gilbert Colletaz (University of Orléans). Current position: assistant professor University Abdelmalek Essâadi, Tanger.
- Sessi Tokpavi (2005-2008). Three Essais on Value-at-Risk. Co-supervisor: Gilbert Colletaz (University of Orléans). Award of the French Association of Finance (AFFI) for the best paper published in the review Finance in 2008. Past positions: associate professor at University Paris Ouest Nanterre (2009-2016), professor at University of Orléans (since 2016).
- Julien Fouquau (2004-2008). Regime-switching models and panel data: from non-linearity to heterogeneity. Co-supervisor: Mélika Ben Salem (University Paris Est). Past position: Associate Professor at Neoma BS, Current position: Professor at ESCP Europe.
Normal
0
21
false
false
false
FR
X-NONE
X-NONE
/* Style Definitions */
table.MsoNormalTable
{mso-style-name: »Tableau Normal »;
mso-tstyle-rowband-size:0;
mso-tstyle-colband-size:0;
mso-style-noshow:yes;
mso-style-priority:99;
mso-style-parent: » »;
mso-padding-alt:0cm 5.4pt 0cm 5.4pt;
mso-para-margin-top:0cm;
mso-para-margin-right:0cm;
mso-para-margin-bottom:10.0pt;
mso-para-margin-left:0cm;
line-height:115%;
mso-pagination:widow-orphan;
font-size:11.0pt;
font-family: »Calibri »,sans-serif;
mso-ascii-font-family:Calibri;
mso-ascii-theme-font:minor-latin;
mso-hansi-font-family:Calibri;
mso-hansi-theme-font:minor-latin;
mso-bidi-font-family: »Times New Roman »;}
Travaux
- Publications dans des revues scientifiques
- Ouvrages et rapports
- Documents de travail et autres publications
- Communications
2024
The Fairness of Credit Scoring Models
In credit markets, screening algorithms aim to discriminate between good-type and bad-type borrowers. However, when doing so, they can also discriminate between individuals sharing a protected attribute (e.g., gender, age, racial origin) and the rest of the population. This can be unintentional and originate from the training data set or from the model itself. We show how to formally test the algorithmic fairness of scoring models and how to identify the variables responsible for any lack of fairness. We then use these variables to optimize the fairness-performance tradeoff. Our framework provides guidance on how algorithmic fairness can be monitored by lenders, controlled by their regulators, improved for the benefit of protected groups, while still maintaining a high level of forecasting accuracy. This paper was accepted by Will Cong, finance. Funding: This work was supported by the Autorité de Contrôle Prudentiel et de Résolution (ACPR) Chair in Regulation and Systemic Risk, the Fintech Chair at Dauphine-PSL University, and the French National Research Agency (ANR) [MLEforRisk ANR-21-CE26-0007, Ecodec ANR-11-LABX-0047, and F-STAR ANR-17-CE26-0007-01]. Supplemental Material: The online appendix and data files are available at https://doi.org/10.1287/mnsc.2022.03888 .
Lien HALComputational Reproducibility in Finance: Evidence from 1,000 Tests
Abstract We analyze the computational reproducibility of more than 1,000 empirical answers to 6 research questions in finance provided by 168 research teams. Running the researchers’ code on the same raw data regenerates exactly the same results only 52% of the time. Reproducibility is higher for researchers with better coding skills and those exerting more effort. It is lower for more technical research questions, more complex code, and results lying in the tails of the distribution. Researchers exhibit overconfidence when assessing the reproducibility of their own research. We provide guidelines for finance researchers and discuss implementable reproducibility policies for academic journals.
Lien HALNonstandard Errors
In statistics, samples are drawn from a population in a data‐generating process (DGP). Standard errors measure the uncertainty in estimates of population parameters. In science, evidence is generated to test hypotheses in an evidence‐generating process (EGP). We claim that EGP variation across researchers adds uncertainty—nonstandard errors (NSEs). We study NSEs by letting 164 teams test the same hypotheses on the same data. NSEs turn out to be sizable, but smaller for more reproducible or higher rated research. Adding peer‐review stages reduces NSEs. We further find that this type of uncertainty is underestimated by participants.
Lien HAL2022
Machine Learning for Credit Scoring: Improving Logistic Regression with Non Linear Decision Tree Effects
In the context of credit scoring, ensemble methods based on decision trees, such as the random forest method, provide better classification performance than standard logistic regression models. However, logistic regression remains the benchmark in the credit risk industry mainly because the lack of interpretability of ensemble methods is incompatible with the requirements of financial regulators. In this paper, we propose a high-performance and interpretable credit scoring method called penalised logistic tree regression (PLTR), which uses information from decision trees to improve the performance of logistic regression. Formally, rules extracted from various short-depth decision trees built with original predictive variables are used as predictors in a penalised logistic regression model. PLTR allows us to capture non-linear effects that can arise in credit scoring data while preserving the intrinsic interpretability of the logistic regression model. Monte Carlo simulations and empirical applications using four real credit default datasets show that PLTR predicts credit risk significantly more accurately than logistic regression and compares competitively to the random forest method
Lien HAL2021
Backtesting Marginal Expected Shortfall and Related Systemic Risk Measures
This paper proposes an original approach for backtesting systemic risk measures. This backtesting approach makes it possible to assess the systemic risk measure forecasts used to identify the financial institutions that contribute the most to the overall risk in the financial system. Our procedure is based on simple tests similar to those generally used to backtest the standard market risk measures such as value-at-risk or expected shortfall. We introduce a concept of violation associated with the marginal expected shortfall (MES), and we define unconditional coverage and independence tests for these violations. We can generalize these tests to any MES-based systemic risk measures such as the systemic expected shortfall (SES), the systemic risk measure (SRISK), or the delta conditional value-at-risk ([Formula: see text]CoVaR). We study their asymptotic properties in the presence of estimation risk and investigate their finite sample performance via Monte Carlo simulations. An empirical application to a panel of U.S. financial institutions is conducted to assess the validity of MES, SRISK, and [Formula: see text]CoVaR forecasts issued from a bivariate GARCH model with a dynamic conditional correlation structure. Our results show that this model provides valid forecasts for MES and SRISK when considering a medium-term horizon. Finally, we propose an early warning system indicator for future systemic crises deduced from these backtests. Our indicator quantifies how much is the measurement error issued by a systemic risk forecast at a given point in time which can serve for the early detection of global market reversals. This paper was accepted by Kay Giesecke, finance.
Lien HAL2019
Pitfalls in systemic-risk scoring
In this paper, we identify several shortcomings in the systemic-risk scoring methodology currently used to identify and regulate Systemically Important Financial Institutions (SIFIs). Using newly-disclosed regulatory data for 119 US and international banks, we show that the current scoring methodology severely distorts the allocation of regulatory capital among banks. We then propose and implement a methodology that corrects for these shortcomings and increases incentives for banks to reduce their risk contributions.
Lien HALMachine learning et nouvelles sources de données pour le scoring de crédit
Résumé non disponible.
Lien HAL2018
Loss Functions for LGD Models Comparison
We propose a new approach for comparing Loss Given Default (LGD) models which is based on loss functions defined in terms of regulatory capital charge. Our comparison method improves the banks' ability to absorb their unexpected credit losses, by penalizing more heavily LGD forecast errors made on credits associated with high exposure and long maturity. We also introduce asymmetric loss functions that only penalize the LGD forecast errors that lead to underestimate the regulatory capital. We show theoretically that our approach ranks models differently compared to the traditional approach which only focuses on LGD forecast errors. We apply our methodology to six competing LGD models using a unique sample of almost 10,000 defaulted credit and leasing contracts provided by an international bank. Our empirical findings clearly show that model rankings based on capital charge losses differ drastically from those based on the LGD loss functions currently used by regulators, banks, and academics.
Lien HAL2017
CoMargin
We present CoMargin, a new methodology to estimate collateral requirements in derivatives central counterparties (CCPs). CoMargin depends on both the tail risk of a given market participant and its interdependence with other participants. Our approach internalizes trading externalities and enhances the stability of CCPs, thus reducing systemic risk concerns. We assess our methodology using proprietary data from the Canadian Derivatives Clearing Corporation that include daily observations of the actual trading positions of all of its members from 2003 to 2011. We show that CoMargin outperforms existing margining systems by stabilizing the probability and minimizing the shortfall of simultaneous margin-exceeding losses.
Lien HALRisk Measure Inference
We propose a bootstrap-based test of the null hypothesis of equality of two firms? conditional Risk Measures (RMs) at a single point in time. The test can be applied to a wide class of conditional risk measures issued from parametric or semi-parametric models. Our iterative testing procedure produces a grouped ranking of the RMs, which has direct application for systemic risk analysis. Firms within a group are statistically indistinguishable form each other, but significantly more risky than the firms belonging to lower ranked groups. A Monte Carlo simulation demonstrates that our test has good size and power properties. We apply the procedure to a sample of 94 U.S. financial institutions using ?CoVaR, MES, and %SRISK. We find that for some periods and RMs, we cannot statistically distinguish the 40 most risky firms due to estimation uncertainty.
Lien HALLa relation firme-analyste explique-t-elle les erreurs de prévision des analystes ?
L'article vérifie dans quelle mesure l'intensité de la relation entre une firme et un analyste financier améliore ou dégrade la précision des prévisions produites par cet analyste sur cette firme. A partir d'un échantillon de prévisions de Bénéfices Par Action (BPA) sur 208 entreprises françaises, nous régressons l'erreur de prévision des analystes sur un ensemble de variables observables. Puis nous décomposons l'effet fixe de la régression et utilisons l'effet couple firme-analyste comme mesure de l'intensité de la relation. On montre qu'un effet couple faible (important) est associé à une erreur de prévision faible (importante), suggérant qu'une relation étroite entre une firme et un analyste tend à biaiser la prévision de ce dernier. Les analystes expérimentés et spécialisés dans le suivi des firmes à forte capitalisation semblent cependant moins sujets à ce biais.
Lien HALWhere the Risks Lie: A Survey on Systemic Risk
We review the extensive literature on systemic risk and connect it to the current regulatory debate. While we take stock of the achievements of this rapidly growing field, we identify a gap between two main approaches. The first one studies different sources of systemic risk in isolation, uses confidential data, and inspires targeted but complex regulatory tools. The second approach uses market data to produce global measures which are not directly connected to any particular theory, but could support a more efficient regulation. Bridging this gap will require encompassing theoretical models and improved data disclosure.
Lien HAL2016
Do We Need High Frequency Data to Forecast Variances?
In this paper we study various MIDAS models for which the future daily variance is directly related to past observations of intraday predictors. Our goal is to determine if there exists an optimal sampling frequency in terms of variance prediction. Via Monte Carlo simulations we show that in a world without microstructure noise, the best model is the one using the highest available frequency for the predictors. However, in the presence of microstructure noise, the use of very high-frequency predictors may be problematic, leading to poor variance forecasts. The empirical application focuses on two highly liquid assets (i.e., Microsoft and S&P 500). We show that, when using raw intraday squared log-returns for the explanatory variable, there is a “high-frequency wall” – or frequency limit – above which MIDAS-RV forecasts deteriorate or stop improving. An improvement can be obtained when using intraday squared log-returns sampled at a higher frequency, provided they are pre-filtered to account for the presence of jumps, intraday diurnal pattern and/or microstructure noise. Finally, we compare the MIDAS model to other competing variance models including GARCH, GAS, HAR-RV and HAR-RV-J models. We find that the MIDAS model – when it is applied on filtered data –provides equivalent or even better variance forecasts than these models. JEL: C22, C53, G12 / KEY WORDS: Variance Forecasting, MIDAS, High-Frequency Data. RÉSUMÉ. Nous considérons dans cet article des modèles de régression MIDAS pour examiner l'influence de la fréquence d'échantillonnage des prédicteurs sur la qualité des prévisions de la volatilité quotidienne. L'objectif principal est de vérifier si l'information incorporée par les prédicteurs à haute fréquence améliore la qualité des précisions de volatilité, et si oui, s'il existe une fréquence d'échantillonnage optimale de ces prédicteurs en termes de prédiction de la variance. Nous montrons, via des simulations Monte Carlo, que dans un monde sans bruit de microstructure, le meilleur modèle est celui qui utilise des prédicteurs à la fréquence la plus élevée possible. Cependant, en présence de bruit de microstructure, l'utilisation des měmes prédicteurs à haute fréquence peut ětre problématique, conduisant à des prévisions pauvres de la variance. L'application empirique se concentre sur deux actifs très liquides (Microsoft et S & P 500). Nous montrons que, lors de l'utilisation des rendements intra-journaliers au carré pour la variable explicative, il y a un « mur à haute fréquence » – ou limite de fréquence – au-delà duquel les prévisions des modèles MIDAS-RV se détériorent ou arrětent de s'améliorer. Une amélioration pourrait ětre obtenue lors de l'utilisation des rendements au carré échantillonnés à une fréquence plus élevée, à condition qu'ils soient préfiltrés pour tenir compte de la présence des sauts, de la saisonnalité intra-journalière et/ou du bruit de microstructure. Enfin, nous comparons le modèle MIDAS à d'autres modèles de variance concurrents, y compris les modèles GARCH, GAS, HAR-RV et HAR-RV-J. Nous constatons que le modèle MIDAS – quand il est appliqué sur des données filtrées – fournit des prévisions de variance équivalentes ou měme meilleures que ces modèles.
Lien HAL2015
A DARE for VaR
This paper introduces a new class of models for the Value-at-Risk (VaR) and Expected Shortfall (ES), called the Dynamic AutoRegressive Expectiles (DARE) models. Our approach is based on a weighted average of expectile-based VaR and ES models, i.e. the Conditional Autoregressive Expectile (CARE) models introduced by Taylor (2008a) and Kuan et al. (2009). First, we briefly present the main non-parametric, parametric and semi-parametric estimation methods for VaR and ES. Secondly, we detail the DARE approach and show how the expectiles can be used to estimate quantile risk measures. Thirdly, we use various backtesting tests to compare the DARE approach to other traditional methods for computing VaR forecasts on the French stock market. Finally, we evaluate the impact of several conditional weighting functions and determine the optimal weights in order to dynamically select the more relevant global quantile model.
Lien HALImplied Risk Exposures
We show how to reverse-engineer banks’ risk disclosures, such as value-at-risk, to obtain an implied measure of their exposures to equity, interest rate, foreign exchange, and commodity risks. Factor implied risk exposures are obtained by breaking down a change in risk disclosure into a market volatility component and a bank-specific risk exposure component. In a study of large US and international banks, we show that (i) changes in risk exposures are negatively correlated with market volatility and (ii) changes in risk exposures are positively correlated across banks, which is consistent with banks exhibiting commonality in trading.
Lien HAL2014
2013
Testing Interval Forecasts: a GMM-Based Approach
This paper proposes a new evaluation framework for interval forecasts. Our model-free test can be used to evaluate interval forecasts and high-density regions, potentially discontinuous and/or asymmetric. Using a simple J-statistic, based on the moments defined by the orthonormal polynomials associated with the binomial distribution, this new approach presents many advantages. First, its implementation is extremely easy. Second, it allows for a separate test for unconditional coverage, independence and conditional coverage hypotheses. Third, Monte Carlo simulations show that for realistic sample sizes our GMM test has good small-sample properties. These results are corroborated by an empirical application on SP500 and Nikkei stock market indexes. It confirms that using this GMM test leads to major consequences for the ex post evaluation of interval forecasts produced by linear versus nonlinear models
Lien HALIs public capital really productive? A methodological reappraisal
We present an evaluation of the main empirical approaches used in the literature to estimate the contribution of public capital stock to growth and private factors' productivity. Based on a simple stochastic general equilibrium model, built as to reproduce the main long-run relations observed in US post-war historical data, we show that the production function approach may not be reliable to estimate this contribution. Our analysis reveals that this approach largely overestimates the public capital elasticity, given the presence of a common stochastic trend shared by all non-stationary inputs.
Lien HALMultivariate Dynamic Probit Models: An Application to Financial Crises Mutation
Forthcoming
Lien HAL2012
Sampling Error and Double Shrinkage Estimation of Minimum Variance Portfolios
Shrinkage estimators of the covariance matrix are known to improve the stability over time of the Global Minimum Variance Portfolio (GMVP), as they are less error-prone. However, the improvement over the empirical covariance matrix is not optimal for small values of n, the estimation sample size. For typical asset allocation problems, with n small, this paper aims at. proposing a new method to further reduce sampling error by shrinking once again traditional shrinkage estimators of the GMVP. First, we show analytically that the weights of any GMVP can be shrunk - within the framework of the ridge regression - towards the ones of the equally-weighted portfolio in order to reduce sampling error. Second, Monte Carlo simulations and empirical applications show that applying our methodology to the GMVP based on shrinkage estimators of the covariance matrix, leads to more stable portfolio weights, sharp decreases in portfolio turnovers, and often statistically lower (resp. higher) out-of-sample variances (resp. Sharpe ratios). These results illustrate that double shrinkage estimation of the GMVP can be beneficial for realistic small estimation sample sizes.
Lien HALHow to Evaluate an Early Warning System? Towards a Unified Statistical Framework for Assessing Financial Crises Forecasting Methods
Forthcoming
Lien HAL2010
What would Nelson and Plosser find had they used panel unit root tests?
In this study, we systemically apply nine recent panel unit root tests to the same fourteen macroeconomic and financial series as those considered in the seminal paper by Nelson and Plosser (1982). The data cover OECD countries from 1950 to 2003. Our results clearly point out the difficulty that applied econometricians would face when they want to get a simple and clear-cut diagnosis with panel unit root tests. We confirm the fact that panel methods must be very carefully used for testing unit roots in macroeconomic or financial panels. More precisely, we find mitigated results under the cross-sectional independence assumption, since the unit root hypothesis is rejected for many macroeconomic variables. When international cross-correlations are taken into account, conclusions depend on the specification of these cross-sectional dependencies. Two groups of tests can be distinguished. The first group tests are based on a dynamic factor structure or an error component model. In this case, the non stationarity of common factors (international business cycles or growth trends) is not rejected, but the results are less clear with respect to idiosyncratic components. The second group tests are based on more general specifications. Their results are globally more favourable to the unit root assumption.
Lien HAL2009
Energy demand models: a threshold panel specification of the 'Kuznets curve
This article proposes an original panel specification of the energy demand model. Based on panel threshold regression models, we derive country-specific and time-specific energy elasticity. We find a fall of the elasticity when the income level increase.
Lien HAL2008
The Feldstein-Horioka Puzzle: a Panel Smooth Transition Regression Approach
Résumé non disponible.
Lien HALThe Feldstein-Horioka Puzzle: a Panel Smooth Transition Regression Approach
Résumé non disponible.
Lien HALThe Feldstein-Horioka Puzzle: a Panel Smooth Transition Regression Approach
Résumé non disponible.
Lien HAL2007
Energy Demand Models: A Threshold Panel Specification of the "Kuznets Curve
Résumé non disponible.
Lien HALThe Feldstein-Horioka Puzzle: a Panel Smooth Transition Regression Approach
Résumé non disponible.
Lien HALCredit Market Disequilibrium in Poland: Can we find what we expect? Non stationarity and the Short Side Rule
Résumé non disponible.
Lien HALUne évaluation des procédures de Backtesting : Tout va pour le mieux dans le meilleur des mondes
Résumé non disponible.
Lien HALUne évaluation des procédures de Backtesting : Tout va pour le mieux dans le meilleur des mondes
Résumé non disponible.
Lien HAL2006
Une Synthèse des Tests de Racine Unitaire sur Données de Panel
Cet article propose une synthèse de la littérature concernant les tests de racine unitaire en panel. Deux principales évolutions peuvent être mises en évidence dans cette voie de recherche depuis les travaux fondateurs de Levin et Lin (1992). D'une part, on a pu assister depuis la fin des années 90 à une évolution tendant à prendre en compte une hétérogénéité des propriétés dynamiques des séries étudiées, avec notamment les travaux d'Im, Pesaran et Shin (1997) et de Maddala et Wu (1999). D'autre part, un second type de développements récents dans cette littérature tend à introduire une dichotomie entre deux générations de tests : la première génération repose sur une hypothèse d'indépendance entre les individus, ce qui apparaît peu plausible notamment dans le cas de certaines applications macro-économiques. La seconde génération, actuellement en plein développement, intègre diverses formes possibles de dépendances inter-individuelles (Bai et Ng (2001), Phillips et Sul (2003a), Moon et Perron (2004), Choi (2002), Pesaran (2003) et Chang (2002)). Ces deux générations de tests sont présentées dans cette revue de la littérature.
Lien HALNetworks Effects in the Productivity of Infrastructures in Developing Countries
Résumé non disponible.
Lien HAL2005
Un Test Simple de l'Hypothèse de Non Causalité dans un Modèle de Panel Hétérogène
Résumé non disponible.
Lien HAL1999
2024
The Economics of Computational Reproducibility
We investigate why economics displays a relatively low level of computational reproducibility. We first study the benefits and costs of reproducibility for readers, authors, and academic journals. Second, we show that the equilibrium level of reproducibility may be suboptimally low due to three market failures: a competitive bottleneck effect due to the competition among journals to attract authors, the public good dimension of reproducibility, and the positive externalities of reproducibility outside academia. Third, we discuss different policies to address these market failures and move out of a low reproducibility equilibrium. In particular, we show that coordination among journals could reduce by half the cost of verifying the reproducibility of accepted papers.
Lien HAL2023
Machine Learning and IRB Capital Requirements
This survey proposes a theoretical and practical reflection on the use of machine learning methods in the context of the Internal Ratings Based (IRB) approach to banks' capital requirements. While machine learning is still rarely used in the regulatory domain (IRB, IFRS 9, stress tests), recent discussions initiated by the European Banking Authority suggest that this may change in the near future. While technically complex, this subject is crucial given growing concerns about the potential financial instability caused by the banks' use of opaque internal models. Conversely, for their proponents, machine learning models offer the prospect of better measurement of credit risk and enhancing financial inclusion. This survey yields several conclusions and recommendations regarding (i) the accuracy of risk parameter estimations, (ii) the level of regulatory capital, (iii) the trade-off between performance and interpretability, (iv) international banking competition, and (v) the governance and operational risks of machine learning models.
Lien HAL2022
Explainable Performance
We introduce the XPER (eXplainable PERformance) methodology to measure the specific contribution of the input features to the predictive or economic performance of a model. Our methodology offers several advantages. First, it is both model-agnostic and performance metric-agnostic. Second, XPER is theoretically founded as it is based on Shapley values. Third, the interpretation of the benchmark, which is inherent in any Shapley value decomposition, is meaningful in our context. Fourth, XPER is not plagued by model specification error, as it does not require re-estimating the model. Fifth, it can be implemented either at the model level or at the individual level. In an application based on auto loans, we find that performance can be explained by a surprisingly small number of features, XPER decompositions are rather stable across metrics, yet some feature contributions switch sign across metrics. Our analysis also shows that explaining model forecasts and model performance are two distinct tasks.
Lien HALReproducibility of Empirical Results: Evidence from 1,000 Tests in Finance
We analyze the computational reproducibility of more than 1,000 empirical answers to six research questions in finance provided by 168 international research teams. Running the original researchers’ code on the same raw data regenerates exactly the same results only 52% of the time. Reproducibility is higher for researchers with better coding skills and for those exerting more effort. It is lower for more technical research questions, more complex code, and for results lying in the tails of the results distribution. Neither researcher seniority, nor peer-review ratings appear to be related to the level of reproducibility. Moreover, researchers exhibit strong overconfidence when assessing the reproducibility of their own research. We provide guidelines for finance researchers and discuss several implementable reproducibility policies for academic journals.
Lien HAL2021
The Fairness of Credit Scoring Models
In credit markets, screening algorithms discriminate between good-type and bad-type borrowers. This is their raison d’être. However, by doing so, they also often discriminate between individuals sharing a protected attribute (e.g. gender, age, race) and the rest of the population. In this paper, we show how to test (1) whether there exists a statistical significant difference in terms of rejection rates or interest rates, called lack of fairness, between protected and unprotected groups and (2) whether this difference is only due to credit worthiness. When condition (2) is not met, the screening algorithm does not comply with the fair-lending principle and can be qualified as illegal. Our framework provides guidance on how algorithmic fairness can be monitored by lenders, controlled by their regulators, and improved for the benefit of protected groups.
Lien HALMachine Learning or Econometrics for Credit Scoring: Let's Get the Best of Both Worlds
In the context of credit scoring, ensemble methods based on decision trees, such as the random forest method, provide better classification performance than standard logistic regression models. However, logistic regression remains the benchmark in the credit risk industry mainly because the lack of interpretability of ensemble methods is incompatible with the requirements of financial regulators. In this paper, we pro- pose to obtain the best of both worlds by introducing a high-performance and interpretable credit scoring method called penalised logistic tree regression (PLTR), which uses information from decision trees to improve the performance of logistic regression. Formally, rules extracted from various short-depth decision trees built with pairs of predictive variables are used as predictors in a penalised logistic regression model. PLTR allows us to capture non-linear effects that can arise in credit scoring data while preserving the intrinsic interpretability of the logistic regression model. Monte Carlo simulations and empirical applications using four real credit default datasets show that PLTR predicts credit risk significantly more accurately than logistic regression and compares competitively to the random forest method. JEL Classification: G10 C25, C53
Lien HAL2020
Backtesting Marginal Expected Shortfall and Related Systemic Risk Measures
Résumé non disponible.
Lien HALReproducibility Certification in Economics Research
Reproducibility is key for building trust in research, yet it is not widespread in economics. We show how external certification can improve reproducibility in economics research. Such certification can be conducted by a trusted third party or agency, which formally tests whether a given result is indeed generated by the code and data used by a researcher. This additional validation step significantly enriches the peer-review process, without adding an extra burden on journals or unduly lengthening the publication process. We show that external certification can accommodate research based on confidential data. Lastly, we present an actual example of external certification.
Lien HAL2019
Machine Learning et nouvelles sources de données pour le scoring de crédit
Dans cet article, nous proposons une réflexion sur l’apport des techniques d’apprentissage automatique (Machine Learning) et des nouvelles sources de données (New Data) pour la modélisation du risque de crédit. Le scoring de crédit fut historiquement l’un des premiers champs d’application des techniques de Machine Learning. Aujourd’hui, ces techniques permettent d’exploiter de « nouvelles » données rendues disponibles par la digitalisation de la relation clientèle et les réseaux sociaux. La conjonction de l’émergence de nouvelles méthodologies et de nouvelles données a ainsi modifié de façon structurelle l’industrie du crédit et favorisé l’émergence de nouveaux acteurs. Premièrement, nous analysons l’apport des algorithmes de Machine Learning à ensemble d’information constant. Nous montrons qu’il existe des gains de productivité liés à ces nouvelles approches mais que les gains de prévision du risque de crédit restent en revanche modestes. Deuxièmement, nous évaluons l’apport de cette « datadiversité », que ces nouvelles données soient exploitées ou non par des techniques de Machine Learning. Il s’avère que certaines de ces données permettent de révéler des signaux faibles qui améliorent sensiblement la qualité de l’évaluation de la solvabilité des emprunteurs. Au niveau microéconomique, ces nouvelles approches favorisent l’inclusion financière et l’accès au crédit des emprunteurs les plus fragiles. Cependant, le Machine Learning appliqué à ces données peut aussi conduire à des biais et à des phénomènes de discrimination.
Lien HALA Theoretical and Empirical Comparison of Systemic Risk Measures
We derive several popular systemic risk measures in a common framework and show that they can be expressed as transformations of market risk measures (e.g. beta). We also derive conditions under which the different measures lead to similar rankings of systemically important financial institutions (SIFIs). In an empirical analysis of US financial institutions, we show that (1) different systemic risk measures identify different SIFIs and that (2) firm rankings based on systemic risk estimates mirror rankings obtained by sorting firms on market risk or liabilities. One-factor linear models explain most of the variability of the systemic risk estimates, which indicates that systemic risk measures fall short in capturing the multiple facets of systemic risk.
Lien HAL2018
Loss functions for LGD model comparison
We propose a new approach for comparing Loss Given Default (LGD) models which is based on loss functions defined in terms of regulatory capital charge. Our comparison method improves the banks' ability to absorb their unexpected credit losses, by penalizing more heavily LGD forecast errors made on credits associated with high exposure and long maturity. We also introduce asymmetric loss functions that only penalize the LGD forecast errors that lead to underestimate the regulatory capital. We show theoretically that our approach ranks models differently compared to the traditional approach which only focuses on LGD forecast errors. We apply our methodology to six competing LGD models using a sample of almost 10,000 defaulted credit and leasing contracts provided by an international bank. Our empirical findings clearly show that models' rankings based on capital charge losses differ from those based on the LGD loss functions currently used by regulators, banks, and academics.
Lien HAL2017
Pitfalls in Systemic-Risk Scoring
We identify several shortcomings in the systemic-risk scoring methodology currently used to identify and regulate Systemically Important Financial Institutions (SIFIs). Using newly-disclosed regulatory data for 119 US and international banks, we show that the current scoring methodology severely distorts the allocation of regulatory capital among banks. We then propose and implement a methodology that corrects for these short-comings and increases incentives for banks to reduce their risk contributions. Unlike the current scores, our adjusted scores are mainly driven by risk indicators directly under the control of the regulated bank and not by factors that are exogenous to the bank, such as exchange rates or other banks' actions.
Lien HAL2015
CoMargin
We present CoMargin, a new methodology to estimate collateral requirements in derivatives central counterparties (CCPs). CoMargin depends on both the tail risk of a given market participant and its interdependence with other participants. Our approach internalizes trading externalities and enhances the stability of CCPs, thus, reducing systemic risk concerns. We assess our methodology using proprietary data from the Canadian Derivatives Clearing Corporation that include daily observations of the actual trading positions of all of its members from 2003 to 2011. We show that CoMargin outperforms existing margining systems by stabilizing the probability and minimizing the shortfall of simultaneous margin-exceeding losses.
Lien HALWhere the Risks Lie: A Survey on Systemic Risk
We review the extensive literature on systemic risk and connect it to the current regulatory debate. While we take stock of the achievements of this rapidly growing field, we identify a gap between two main approaches. The first one studies different sources of systemic risk in isolation, uses confidential data, and inspires targeted but complex regulatory tools. The second approach uses market data to produce global measures which are not directly connected to any particular theory, but could support a more efficient regulation. Bridging this gap will require encompassing theoretical models and improved data disclosure.
Lien HALWhere the Risks Lie: A Survey on Systemic Risk
We review the extensive literature on systemic risk and connect it to the current regulatory debate. While we take stock of the achievements of this rapidly growing field, we identify a gap between two main approaches. The first one studies different sources of systemic risk in isolation, uses confidential data, and inspires targeted but complex regulatory tools. The second approach uses market data to produce global measures which are not directly connected to any particular theory, but could support a more efficient regulation. Bridging this gap will require encompassing theoretical models and improved data disclosure.
Lien HALRisk Measure Inference
We propose a bootstrap-based test of the null hypothesis of equality of two firms' conditional Risk Measures (RMs) at a single point in time. The test can be applied to a wide class of conditional risk measures issued from parametric or semi-parametric models. Our iterative testing procedure produces a grouped ranking of the RMs which has direct application for systemic risk analysis. A Monte Carlo simulation demonstrates that our test has good size and power properties. We propose an application to a sample of U.S. financial institutions using CoVaR, MES, and SRISK, and conclude that only SRISK can be estimated with enough precision to allow for meaningful ranking.
Lien HAL2014
Do We Need Ultra-High Frequency Data to Forecast Variances?
In this paper we study various MIDAS models in which the future daily variance is directly related to past observations of intraday predictors. Our goal is to determine if there exists an optimal sampling frequency in terms of volatility prediction. Via Monte Carlo simulations we show that in a world without microstructure noise, the best model is the one using the highest available frequency for the predictors. However, in the presence of microstructure noise, the use of ultra high-frequency predictors may be problematic, leading to poor volatility forecasts. In the application, we consider two highly liquid assets (i.e., Microsoft and S&P 500). We show that, when using raw intraday squared log-returns for the explanatory variable, there is a "high-frequency wall" or frequency limit above which MIDAS-RV forecasts deteriorate. We also show that an improvement can be obtained when using intraday squared log-returns sampled at a higher frequency, provided they are pre-filtered to account for the presence of jumps, intraday periodicity and/or microstructure noise. Finally, we compare the MIDAS model to other competing variance models including GARCH, GAS, HAR-RV and HAR-RV-J models. We find that the MIDAS model provides equivalent or even better variance forecasts than these models, when it is applied on filtered data.
Lien HALImplied Risk Exposures
We show how to reverse-engineer banks' risk disclosures, such as Value-at-Risk, to obtain an implied measure of their exposures to equity, interest rate, foreign exchange, and commodity risks. Factor Implied Risk Exposures (FIRE) are obtained by breaking down a change in risk disclosure into a market volatility component and a bank-specific risk exposure component. In a study of large US and international banks, we show that (1) changes in risk exposures are negatively correlated with market volatility and (2) changes in risk exposures are positively correlated across banks, which is consistent with banks exhibiting commonality in trading.
Lien HALThe Counterparty Risk Exposure of ETF Investors
As most Exchange-Traded Funds (ETFs) engage in securities lending or are based on total return swaps, they expose their investors to counterparty risk. In this paper, we estimate empirically such risk exposures for a sample of physical and swap-based funds. We find that counterparty risk exposure is higher for swap-based ETFs, but that investors are compensated for bearing this risk. Using a difference-in-differences specification, we uncover that ETF flows respond significantly to changes in counter-party risk. Finally, we show that switching to an optimal collateral portfolio leads to substantial reduction in counterparty risk exposure.
Lien HAL2013
Systemic Risk Score: A Suggestion
In this paper, we identify several shortcomings in the systemic-risk scoring methodology currently used to identify and regulate Systemically Important Financial Institutions (SIFIs). Using newly-disclosed regulatory data for 119 US and international banks, we show that the current scoring methodology severely distorts the allocation of regulatory capital among banks. We then propose and implement a methodology that corrects for these shortcomings and increases incentives for banks to reduce their risk contributions.
Lien HALHigh-Frequency Risk Measures
This paper proposes intraday High Frequency Risk (HFR) measures for market risk in the case of irregularly spaced high-frequency data. In this context, we distinguish three concepts of value-at-risk (VaR): the total VaR, the marginal (or per-time-unit) VaR, and the instantaneous VaR. Since the market risk is obviously related to the duration between two consecutive trades, these measures are completed with a duration risk measure, i.e., the time-at-risk (TaR). We propose a forecasting procedure for VaR and TaR for each trade or other market microstructure event. We perform a backtesting procedure specifically designed to assess the validity of the VaR and TaR forecasts on irregularly spaced data. The performance of the HFR measure is illustrated in an empirical application for two stocks (Bank of America and Microsoft) and an exchange-traded fund (ETF) based on Standard and Poor's (the S&P) 500 index. We show that the intraday HFR forecasts accurately capture the volatility and duration dynamics for these three assets.
Lien HALSystemic Risk Score: A Suggestion
We identify a potential bias in the methodology disclosed in July 2013 by the Basel Committee on Banking Supervision (BCBS) for identifying systemically important financial banks. Contrary to the original objective, the relative importance of the five categories of risk importance (size, cross-jurisdictional activity, interconnectedness, substitutability/financial institution infrastructure, and complexity) may not be equal and the resulting systemic risk scores are mechanically dominated by the most volatile categories. In practice, this bias proved to be serious enough that the substitutability category had to be capped by the BCBS. We show that the bias can be removed by simply standardizing each input prior to computing the systemic risk scores.
Lien HALDoes the firm-analyst relationship matter in explaining analysts' earnings forecast errors?
We study whether financial analysts' concern for preserving good relationships with firms' managers motivates them to issue pessimistic or optimistic forecasts. Based on a dataset of one-yearahead EPS forecasts issued by 4 648 analysts concerning 241 French firms (1997-2007), we regress the analysts' forecast accuracy on its unintentional determinants. We then decompose the fixed effect of the regression and we use the firm-analyst pair effect as a measure of the intensity of the firm-analyst relationship. We find that a low (high) firm-analyst pair effect is associated with a low (high) forecast error. This observation suggests that pessimism and optimism result from the analysts' concern for cultivating their relationship with the firm's management.
Lien HALA Theoretical and Empirical Comparison of Systemic Risk Measures
We derive several popular systemic risk measures in a common framework and show that they can be expressed as transformations of market risk measures (e.g., beta). We also derive conditions under which the different measures lead to similar rankings of systemically important financial institutions (SIFIs). In an empirical analysis of US financial institutions, we show that (1) different systemic risk measures identify different SIFIs and that (2) firm rankings based on systemic risk estimates mirror rankings obtained by sorting firms on market risk or liabilities. One-factor linear models explain most of the variability of the systemic risk estimates, which indicates that systemic risk measures fall short in capturing the multiple facets of systemic risk.
Lien HAL2012
Margin Backtesting
This paper presents a validation framework for collateral requirements or margins on a derivatives exchange. It can be used by investors, risk managers, and regulators to check the accuracy of a margining system. The statistical tests presented in this study are based either on the number, frequency, magnitude, or timing of margin exceedances, which are defined as situations in which the trading loss of a market participant exceeds his or her margin. We also propose an original way to validate globally the margining system by aggregating individual backtesting statistics obtained for each market participant.
Lien HALThe Risk Map: A New Tool for Validating Risk Models
This paper presents a new method to validate risk models: the Risk Map. This method jointly accounts for the number and the magnitude of extreme losses and graphically summarizes all information about the performance of a risk model. It relies on the concept of a super exception, which is de.ned as a situation in which the loss exceeds both the standard Value-at-Risk (VaR) and a VaR de.ned at an extremely low coverage probability. We then formally test whether the sequences of exceptions and super exceptions are rejected by standard model validation tests. We show that the Risk Map can be used to validate market, credit, operational, or systemic risk estimates (VaR, stressed VaR, expected shortfall, and CoVaR) or to assess the performance of the margin system of a clearing house.
Lien HALMultivariate Dynamic Probit Models: An Application to Financial Crises Mutation
In this paper we propose a multivariate dynamic probit model. Our model can be considered as a non-linear VAR model for the latent variables associated with correlated binary time-series data. To estimate it, we implement an exact maximum-likelihood approach, hence providing a solution to the problem generally encountered in the formulation of multivariate probit models. Our framework allows us to apprehend dynamics and causality in several ways. Furthermore, we propose an impulse-response analysis for such models. An empirical application on three nancial crises is nally proposed.
Lien HALBacktesting Value-at-Risk: From Dynamic Quantile to Dynamic Binary Tests
In this paper we propose a new tool for backtesting that examines the quality of Value-at- Risk (VaR) forecasts. To date, the most distinguished regression-based backtest, proposed by Engle and Manganelli (2004), relies on a linear model. However, in view of the di- chotomic character of the series of violations, a non-linear model seems more appropriate. In this paper we thus propose a new tool for backtesting (denoted DB) based on a dy- namic binary regression model. Our discrete-choice model, e.g. Probit, Logit, links the sequence of violations to a set of explanatory variables including the lagged VaR and the lagged violations in particular. It allows us to separately test the unconditional coverage, the independence and the conditional coverage hypotheses and it is easy to implement. Monte-Carlo experiments show that the DB test exhibits good small sample properties in realistic sample settings (5% coverage rate with estimation risk). An application on a portfolio composed of three assets included in the CAC40 market index is nally proposed.
Lien HALHow to evaluate an Early Warning System ?
This paper proposes an original and uni ed toolbox to evaluate nancial crisis Early Warning Systems (EWS). It presents four main advantages. First, it is a model free method which can be used to asses the forecasts issued from di erent EWS (probit, logit, markov switching models, or combinations of models). Second, this toolbox can be applied to any type of crisis EWS (currency, banking, sovereign debt, etc.). Third, it does not only provide various criteria to evaluate the (absolute) validity of EWS forecasts but also proposes some tests to compare the relative performance of alternative EWS. Fourth, our toolbox can be used to evaluate both in-sample and out-of-sample forecasts. Applied to a logit model for twelve emerging countries we show that the yield spread is a key variable to predict currency crises exclusively for South-Asian countries. Besides, the optimal cut-o correctly allows us to identify now on average more than 2/3 of the crisis and calm periods.
Lien HALExtreme Financial Cycles
This paper proposes a new approach to date extreme financial cycles. Elaborating on recent methods in extreme value theory, it elaborates an extension of the famous calculus rule to detect extreme peaks and troughs. Applied on United-States stock market since 1871, it leads to a dating of these exceptional events and calls for adequate economic policies in order to tackle them.
Lien HALRunMyCode.org: a novel dissemination and collaboration platform for executing published computational results
We believe computational science as practiced today suffers from a growing credibility gap - it is impossible toreplicate most of the computational results presented at conferences or published in papers today. We argue that this crisis can be addressed by the open availability of the code and data that generated the results, in other words practicing reproducible computational science. In this paper we present a new computational infrastructure called RunMyCode.org that is designed to support published articles by providing a dissemination platform for the code and data that generated the their results. Published articles are given a companion webpage on the RunMyCode.org website from which a visitor can both download the associated code and data, and execute the code in the cloud directly through the RunMyCode.org website. This permits results to be verified through the companion webpage or on a user's local system. RunMyCode.org also permits a user to upload their own data to the companion webpage to check the code by running it on novel datasets. Through the creation of "coder pages" for each contributor to RunMyCode.org, we seek to facilitate social network-like interaction. Descriptive information appears on each coder page, including demographic data and other companion pages to which they made contributions. In this paper we motivate the rationale and functionality of RunMyCode.org and outline a vision of its future.
Lien HALIs Public Capital Really Productive? A Methodological Reappraisal
We present an evaluation of the main empirical approaches used in the literature to estimate the contribution of public capital stock to growth and private factors' productivity. Based on a simple stochastic general equilibrium model, built as to reproduce the main long-run relations observed in US post-war historical data, we show that the production function approach may not be reliable to estimate this contribution. Our analysis reveals that this approach largely overestimates the public capital elasticity, given the presence of a common stochastic trend shared by all non-stationary inputs.
Lien HALTesting for Granger Non-causality in Heterogeneous Panels
This paper proposes a very simple test of Granger (1969) non-causality for hetero- geneous panel data models. Our test statistic is based on the individual Wald statistics of Granger non causality averaged across the cross-section units. First, this statistic is shown to converge sequentially to a standard normal distribution. Second, the semi- asymptotic distribution of the average statistic is characterized for a fixed T sample. A standardized statistic based on an approximation of the moments of Wald statistics is hence proposed. Third, Monte Carlo experiments show that our standardized panel statistics have very good small sample properties, even in the presence of cross-sectional dependence.
Lien HAL2011
A Theoretical and Empirical Comparison of Systemic Risk Measures: MES versus CoVaR
We derive several popular systemic risk measures in a common framework and show that they can be expressed as transformations of market risk measures (e.g., beta). We also derive conditions under which the different measures lead to similar rankings of systemically important financial institutions (SIFIs). In an empirical analysis of US financial institutions, we show that (1) different systemic risk measures identify different SIFIs and that (2) firm rankings based on systemic risk estimates mirror rankings obtained by sorting firms on market risk or liabilities. One-factor linear models explain most of the variability of the systemic risk estimates, which indicates that systemic risk measures fall short in capturing the multiple facets of systemic risk.
Lien HALDoes soft information matter for financial analysts' forecasts? A gravity model approach
We study whether the financial analysts' concern to maintain good relationships with firms' managers in order to preserve their access to 'soft' qualitative information entice them to issue pessimistic or optimistic forecasts. We use a gravity model approach to firmsanalysts relationships and propose a measure of soft information. Our database contains the one-year ahead EPS forecasts issued by 4 648 analysts about 241 French firms (1997-2007). We find that a low (high) pair-effect is associated with a low (high) forecast error. This suggests that pessimism and optimism result from analysts' concern to preserve access to soft information released by managers.
Lien HALTesting interval forecasts: a GMM-based approach
This paper proposes a new evaluation framework for interval forecasts. Our model free test can be used to evaluate intervals forecasts and High Density Regions, potentially discontinuous and/or asymmetric. Using a simple J-statistic, based on the moments de ned by the orthonormal polynomials associated with the Binomial distribution, this new approach presents many advantages. First, its implementation is extremely easy. Second, it allows for a separate test for unconditional coverage, independence and conditional coverage hypotheses. Third, Monte-Carlo simulations show that for realistic sample sizes, our GMM test has good small-sample properties. These results are corroborated by an empirical application on SP500 and Nikkei stock market indexes. It con rms that using this GMM test leads to major consequences for the ex-post evaluation of interval forecasts produced by linear versus nonlinear models.
Lien HAL2010
Un MEDAF à plusieurs moments réalisés
Cet article généralise l'approche de Bollerslev et Zhang (2003) qui consiste à utiliser des mesures et co-mesures de risque "réalisées" pour l'estimation des sensibilités dans les modèles d'évaluation des actifs financiers. Nous proposons ici d'étendre cette approche en introduisant les moments d'ordre supérieur et développons des méthodologies d'estimation visant à neutraliser les erreurs de spécification et de modèle. A partir d'une base de données des prix de haute fréquence du marché français des actions, nous établissons que le recours à des mesures réalisées d'ordre supérieur contribue à améliorer l'ajustement global aux données de marché.
Lien HAL2008
Backtesting Value-at-Risk: A GMM Duration-Based Test
This paper proposes a new duration-based backtesting procedure for VaR forecasts. The GMM test framework proposed by Bontemps (2006) to test for the distributional assumption (i.e. the geometric distribution) is applied to the case of the VaR forecasts validity. Using simple J-statistic based on the moments defined by the orthonormal polynomials associated with the geometric distribution, this new approach tackles most of the drawbacks usually associated to duration based backtesting procedures. First, its implementation is extremely easy. Second, it allows for a separate test for unconditional coverage, independence and conditional coverage hypothesis (Christoffersen, 1998). Third, feasibility of the tests is improved. Fourth, Monte-Carlo simulations show that for realistic sample sizes, our GMM test outperforms traditional duration based test. An empirical application for Nasdaq returns confirms that using GMM test leads to major consequences for the ex-post evaluation of the risk by regulation authorities. Without any doubt, this paper provides a strong support for the empirical application of duration-based tests for VaR forecasts.
Lien HALFinancial Development and Growth: A Re-Examination using a Panel Granger Causality Test
In this paper we investigate the causal relationship between financial development and economic growth. We use an innovative econometric method which is based on a panel test of the Granger non causality hypothesis. We implement various tests with a sample of 63 industrial and developing countries over the 1960-1995 and 1960-2000 periods. We use three standard indicators of financial development. The results provide support for a robust causality relationship from economic growth to the financial development. On the contrary, the non causality hypothesis from financial development indicators to economic growth can not be rejected in most of the cases. However, these results only imply that, if such a relationship exists, it can not be easily identified in a simply bi-variate Granger causality test.
Lien HALThreshold Effects in the Public Capital Productivity: An International Panel Smooth Transition Approach
Using a nonlinear panel data model, we examine the threshold effects in the productivity of the public capital stocks for a panel of 21 OECD countries observed over 1965-2001. Using the so-called "augmented production function" approach, we estimate various specifications of a Panel Smooth Threshold Regression (PSTR) model recently developed by Gonzalez, Teräsvirta and Van Dijk (2004). One of our main results is the existence of strong threshold effects in the relationship between output and private and public inputs: whatever the transition mechanism specified, tests strongly reject the linearity assumption. Moreover, this model allows cross-country heterogeneity and time instability of the productivity without specification of an ex-ante classification over individuals. Consequently, it is possible to give estimates of productivity coefficients for both private and public capital stocks at any time and for all the countries. Finally we proposed estimates of individual time varying elasticities that are much more reasonable than those previously published.
Lien HALPublic Spending Efficiency: an Empirical Analysis for Seven Fast Growing Countries
Résumé non disponible.
Lien HALEstimates of Government Net Capital Stocks for 26 Developing Countries, 1970-2002
Résumé non disponible.
Lien HAL2007
The Feldstein-Horioka Puzzle: a Panel Smooth Transition Regression Approach
This paper proposes an original framework to determine the relative influence of five factors on the Feldstein and Horioka result of OECD countries with a strong saving- investment association. Based on panel threshold regression models, we establish country-specific and time-specific saving retention coefficients for 24 OECD coun- tries over the period 1960-2000. These coefficients are assumed to change smoothly, as a function of five threshold variables, considered as the most important in the literature devoted to the Feldstein and Horioka puzzle. The results show that; de- gree of openness, country size and current account to GDP ratios have the greatest influence on the investment-saving relationship.
Lien HALIrregularly Spaced Intraday Value at Risk (ISIVaR) Models : Forecasting and Predictive Abilities
The objective of this paper is to propose a market risk measure defined in price event time and a suitable backtesting procedure for irregularly spaced data. Firstly, we combine Autoregressive Conditional Duration models for price movements and a non parametric quantile estimation to derive a semi-parametric Irregularly Spaced Intraday Value at Risk (ISIVaR) model. This ISIVaR measure gives two information: the expected duration for the next price event and the related VaR. Secondly, we use a GMM approach to develop a backtest and investigate its finite sample properties through numerical Monte Carlo simulations. Finally, we propose an application to two NYSE stocks.
Lien HALUne Evaluation des Procédures de Backtesting
Dans cet article, nous proposons une démarche originale visant à évaluer la capacité des tests usuels de backtesting à discriminer différentes prévisions de Value at Risk (VaR) ne fournissant pas la même évaluation ex-ante du risque. Nos résultats montrent que, pour un même actif, ces tests conduisent très souvent à ne pas rejeter la validité, au sens de la couverture conditionnelle, de la plupart des six prévisions de VaR étudiées, même si ces dernières sont sensiblement différentes. Autrement dit, toute prévision de VaR a de fortes chances d'être validée par ce type de procédure.
Lien HALSecond Generation Panel Unit Root Tests
This article proposes an overview of the recent developments relating to panel unit root tests. After a brief review of the first generation panel unit root tests, this paper focuses on the tests belonging to the second generation. The latter category of tests is characterized by the rejection of the cross-sectional independence hypothesis. Within this second generation of tests, two main approaches are distinguished. The first one relies on the factor structure approach and includes the contributions of Bai and Ng (2001), Phillips and Sul (2003a), Moon and Perron (2004a), Choi (2002) and Pesaran (2003) among others. The second approach consists in imposing few or none restrictions on the residuals covariance matrix and has been adopted notably by Chang (2002, 2004), who proposed the use of nonlinear instrumental variables methods or the use of bootstrap approaches to solve the nuisanceparameter problem due to cross-sectional dependency.
Lien HALModèles Non Linéaires et Prévisions
Ce rapport propose une synthèse de la littérature sur l'apport des modèles non linéaires en matière de prévision des variables économiques et financières. Il comporte trois parties. La première passe en revue les principales modélisations économétriques non linéaires. La seconde partie est consacrée à la construction des prévisions ponctuelles, des prévisions par intervalle de confiance et des densités de prévisions issues des modèles non linéaires. La troisième partie décrit les principales méthodes de validation de ces différentes formes de prévisions.
Lien HALHow to Estimate Public Capital Productivity?
We propose an evaluation of the main empirical approaches used in the literature to estimate the contribution of public capital stock to growth and private factors' productivity. Our analysis is based on the replication of these approaches on pseudo-samples generated using a stochastic general equilibrium model, built as to reproduce the main long-run relations observed in US post-war historical data. The results suggest that the production function approach may not be reliable to estimate this contribution. In our model, this approach largely overestimates the public capital elasticity, given the presence of a common stochastic trend shared by all non-stationary inputs
Lien HALWhat would Nelson and Plosser find had they used panel unit root tests?
In this study, we systemically apply nine recent panel unit root tests to the same fourteen macroeconomic and financial series as those considered in the seminal paper by Nelson and Plosser (1982). The data cover OECD countries from 1950 to 2003. Our results clearly point out the difficulty that applied econometricians would face when they want to get a simple and clear-cut diagnosis with panel unit root tests. We confirm the fact that panel methods must be very carefully used for testing unit roots in macroeconomic or financial panels. More precisely, we find mitigated results under the cross-sectional independence assumption, since the unit root hypothesis is rejected for many macroeconomic variables. When international cross-correlations are taken into account, conclusions depend on the specification of these cross-sectional dependencies. Two groups of tests can be distinguished. The first group tests are based on a dynamic factor structure or an error component model. In this case, the non stationarity of common factors (international business cycles or growth trends) is not rejected, but the results are less clear with respect to idiosyncratic components. The second group tests are based on more general specifications. Their results are globally more favourable to the unit root assumption.
Lien HALIrregularly Spaced Intraday Value at Risk (ISIVaR) Models: Forecasting and Predictive Abilities
Résumé non disponible.
Lien HALIrregularly Spaced Intraday Value at Risk (ISIVaR) Models Forecasting and Predictive Abilities
Résumé non disponible.
Lien HALIrregularly Spaces Intraday Value-at-Risk (ISIVaR) Models: Forecasting and Predictive Abilities
Résumé non disponible.
Lien HAL2006
The Feldstein-Horioka Puzzle : a Panel Smooth Transition Regression Approach
Résumé non disponible.
Lien HALThe Feldstein-Horioka Puzzle : a Panel Smooth Transition Regression Approach
Résumé non disponible.
Lien HALThe Feldstein-Horioka Puzzle : a Panel Smooth Transition Regression Approach
Résumé non disponible.
Lien HALUne synthèse des tests de cointégration sur données de panel
L'objet de ce papier est de dresser un panorama complet de la littérature relative aux tests de cointégration sur données de panel. Après un exposé des concepts spécifiques à la cointégration en panel, sont ainsi présentés les tests de l'hypothèse nulle d'absence de cointégration (tests de Pedroni (1995, 1997, 1999, 2003), Kao (1999), Bai et Ng (2001) et Groen et Kleibergen (2003)) ainsi que le test de McCoskey et Kao (1998) reposant sur l'hypothèse nulle de cointégration. Quelques éléments relatifs à l'inférence et l'estimation de systèmes cointégrés sont également fournis.
Lien HALBacktesting VaR Accuracy: A New Simple Test
This paper proposes a new test of Value at Risk (VaR) validation. Our test exploits the idea that the sequence of VaR violations (Hit function) - taking value 1-α, if there is a violation, and -α otherwise - for a nominal coverage rate α verifies the properties of a martingale difference if the model used to quantify risk is adequate (Berkowitz et al., 2005). More precisely, we use the Multivariate Portmanteau statistic of Li and McLeod (1981) - extension to the multivariate framework of the test of Box and Pierce (1970) - to jointly test the absence of autocorrelation in the vector of Hit sequences for various coverage rates considered as relevant for the management of extreme risks. We show that this shift to a multivariate dimension appreciably improves the power properties of the VaR validation test for reasonable sample sizes.
Lien HALThreshold Effects of the Public Capital Productivity : An International Panel Smooth Transition Approach
Using a non linear panel data model we examine the threshold effects in the productivity of the public capital stocks for a panel of 21 OECD countries observed over 1965-2001. Using the so-called "augmented production function" approach, we estimate various specifications of a Panel Smooth Threshold Regression (PSTR) model recently developed by Gonzalez, Teräsvirta and Van Dijk (2004). One of our main results is the existence of strong threshold effects in the relationship between output and private and public inputs : whatever the transition mechanism specified, tests strongly reject the linearity assumption. Moreover this model allows cross-country heterogeneity and time instability of the productivity without specification of an ex-ante classification over individuals. Consequently it is posible to give estimates of productivity coefficients for both private and public capital stocks at any time and for each countries in the sample. Finally we proposed estimates of individual time varying elasticities that are much more reasonable than those previously published.
Lien HALThreshold Effects in the Public Capital Productivity: An International Panel Smooth Transition Approach
Résumé non disponible.
Lien HALThreshold Effects in the Public Capital Productivity: An International Panel Smooth Transition Approach
Résumé non disponible.
Lien HAL2005
The Heterogeneity of Employment Adjustment Accross Japanese Firms. A study Using Panel Data
Résumé non disponible.
Lien HAL2004
2008
Testing Granger causality in Heterogeneous Panel Data Models with Fixed Coefficients
Résumé non disponible.
Lien HALTesting Granger causality in Heterogeneous Panel Data Models with Fixed Coefficients
Résumé non disponible.
Lien HAL2007
Irregularly Spaced Intraday Value-at-Risk (ISIVaR) Models: Forecasting and Predictive Abilities
Résumé non disponible.
Lien HALIrregularly Spaced Intraday Value-at-Risk (ISIVaR) Models: Forecasting and Predictive Abilities
Résumé non disponible.
Lien HALTesting Granger Causality in Heterogeneous Panel Data Model with Fixed Coefficients
Résumé non disponible.
Lien HAL2006
The Feldstein-Horioka Puzzle : a Panel Smooth Transition Regression Approach
Résumé non disponible.
Lien HALThe Feldstein-Horioka Puzzle: a Panel Smooth Transition Regression Approach
Résumé non disponible.
Lien HALThe Feldstein-Horioka Puzzle : a Panel Smooth Transition Regression Approach
Résumé non disponible.
Lien HALThreshold Effects in the Public Capital Productivity: an International Panel Smooth Transition Approach
Résumé non disponible.
Lien HALThreshold Effects of the Public Capital Productivity: an International Panel Smooth Transition Approach
Résumé non disponible.
Lien HALThreshold Effects of the Public Capital Productivity: an International Panel Smooth Transition Approach
Résumé non disponible.
Lien HALThreshold Effects in the Public Capital Productivity: an International Panel Smooth Transition Approach
Résumé non disponible.
Lien HAL2005
Une évaluation des procédures de Backtesting : Tout va pour le mieux dans le meilleur des mondes
Résumé non disponible.
Lien HAL2004